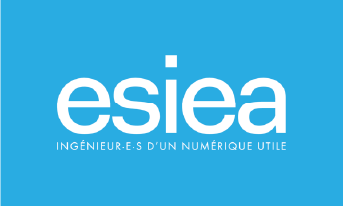
Jeudi 13
9h30 - 11h30
Salle 4
INTERVENTION PROPOSÉE PAR
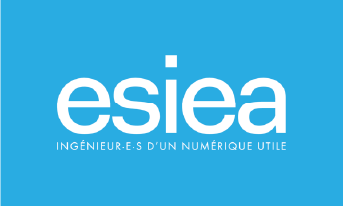
DESCRIPTION
Mobility datasets are crucial for various applications. However, sharing this data raises privacy concerns due
to the sensitive nature of geolocation information. Synthetic
data generation has recently emerged as a promising solution
to protect geo-privacy of trajectory data. Current approaches
rely on having a large set of authentic trajectories collected
from individual users to train generative networks. However, this
assumption proves impractical in many real-world scenarios due
to the sensitive personal information typically embedded within
trajectories. Our approach leverages federated learning to generate privacy-preserving synthetic trajectories without the need
for centralized data collection. Experimental results demonstrate
that our distributed framework effectively produces synthetic
trajectories with distributions comparable to baseline, offering
a privacy-conscious alternative for geo-privacy protection in
mobility datasets.
SPEAKERS
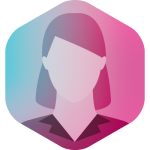
AUTRES INTERVENTIONS
Jeudi 13
15h00 - 15h15
Challenge IA Luminess x ESIEA
Salle des congrès
Jeudi 13
9h30 - 11h30
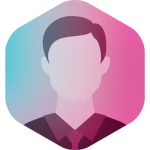
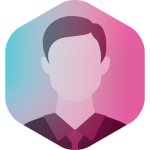
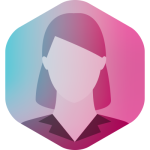
Vision EEG
Salle 4
Jeudi 13
9h30 - 11h30
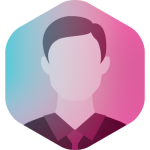
Conception d’un système embarqué de vision par ordinateur avec IA pour la détection e...
Salle 4
Jeudi 13
9h30 - 11h30
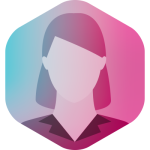
Accurate Hand Contact Detection from RGB Images via Image-to-Image Translation
Salle 4
Jeudi 13
9h30 - 11h30
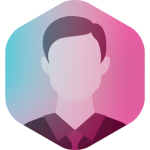
Semantic Retention and Extreme Compression in LLMs: Can We Have Both?
Salle 4
Jeudi 13
9h30 - 11h30
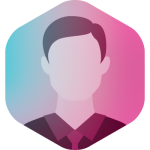
APT cyberattack simulation and intent-based mitigation planning
Salle 4